TBA
November, 25th, 2020
TITLE
Donnez plus de détails sur vos services. Utilisez cette mise en page répétitive pour afficher votre contenu. C'est le moyen idéal pour tenir vos clients informés. Vous souhaitez personnaliser ce texte à votre image ? Glissez-déposez simplement vos éléments comme des images, des liens ou du texte ou connectez les données de votre collection. Donnez plus de détails sur vos services. Utilisez cette mise en page répétitive pour afficher votre
TBA
November, 25th, 2020
TITLE
Donnez plus de détails sur vos services. Utilisez cette mise en page répétitive pour afficher votre contenu. C'est le moyen idéal pour tenir vos clients informés. Vous souhaitez personnaliser ce texte à votre image ? Glissez-déposez simplement vos éléments comme des images, des liens ou du texte ou connectez les données de votre collection. Donnez plus de détails sur vos services. Utilisez cette mise en page répétitive pour afficher votre
TBA
November, 18, 2020
TITLE
Donnez plus de détails sur vos services. Utilisez cette mise en page répétitive pour afficher votre contenu. C'est le moyen idéal pour tenir vos clients informés. Vous souhaitez personnaliser ce texte à votre image ? Glissez-déposez simplement vos éléments comme des images, des liens ou du texte ou connectez les données de votre collection. Donnez plus de détails sur vos services. Utilisez cette mise en page répétitive pour afficher votre
TBA
November, 25th, 2020
TITLE
Donnez plus de détails sur vos services. Utilisez cette mise en page répétitive pour afficher votre contenu. C'est le moyen idéal pour tenir vos clients informés. Vous souhaitez personnaliser ce texte à votre image ? Glissez-déposez simplement vos éléments comme des images, des liens ou du texte ou connectez les données de votre collection. Donnez plus de détails sur vos services. Utilisez cette mise en page répétitive pour afficher votre
TBA
November, 25th, 2020
TITLE
Donnez plus de détails sur vos services. Utilisez cette mise en page répétitive pour afficher votre contenu. C'est le moyen idéal pour tenir vos clients informés. Vous souhaitez personnaliser ce texte à votre image ? Glissez-déposez simplement vos éléments comme des images, des liens ou du texte ou connectez les données de votre collection. Donnez plus de détails sur vos services. Utilisez cette mise en page répétitive pour afficher votre
TBA
November, 18, 2020
TITLE
Donnez plus de détails sur vos services. Utilisez cette mise en page répétitive pour afficher votre contenu. C'est le moyen idéal pour tenir vos clients informés. Vous souhaitez personnaliser ce texte à votre image ? Glissez-déposez simplement vos éléments comme des images, des liens ou du texte ou connectez les données de votre collection. Donnez plus de détails sur vos services. Utilisez cette mise en page répétitive pour afficher votre
TBA
November, 18, 2020
TITLE
Donnez plus de détails sur vos services. Utilisez cette mise en page répétitive pour afficher votre contenu. C'est le moyen idéal pour tenir vos clients informés. Vous souhaitez personnaliser ce texte à votre image ? Glissez-déposez simplement vos éléments comme des images, des liens ou du texte ou connectez les données de votre collection. Donnez plus de détails sur vos services. Utilisez cette mise en page répétitive pour afficher votre

David Kleinfeld
UCSD
October 11, 2023
Exceptionally the talk will start at 11:15 am ET
Vasomotor dynamics: Measuring, modeling, and understanding the other network in the brain
Much as Santiago Ramón y Cajal is the godfather of neuronal computation, which occurs among neurons that communicate predominantly via threshold logic, Camillo Golgi is the inadvertent godfather of neurovascular signaling, in which the endothelial cells that form the lumen of blood vessels communicate via electrodiffusion as well as threshold logic. I will address questions that define spatiotemporal patterns of constriction and dilation that develop across the network of cortical vasculature: First - is there a common topology and geometry of brain vasculature (our work)? Second - what mechanisms govern neuron-to-vessel and vessel-to-vessel signaling (work of Mark Nelson at U Vermont)? Last - what is the nature of competition among arteriole smooth muscle oscillators and the underlying neuronal drive (our work)? This answers to these questions bear on fundamental aspects of brain science as well as practical issues, including the relation of fMRI signals to neuronal activity and the impact of vascular dysfunction on cognition. Challenges and opportunities for experimentalists and theorists alike will be discussed.
Alaa Ahmed
University of Colorado, Boulder
October 18, 2023
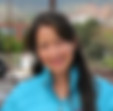
A unifying framework for movement control and decision making
To understand subjective evaluation of an option, various disciplines have quantified the interaction between reward and effort during decision making, producing an estimate of economic utility, namely the subject ‘goodness’ of an option. However, those same variables that affect the utility of an option also influence the vigor (speed) of movements to acquire it. To better understand this, we have developed a mathematical framework demonstrating how utility can influence not only the choice of what to do, but also the speed of the movement follows. I will present results demonstrating that expectation of reward increases speed of saccadic eye and reaching movements, whereas expectation of effort expenditure decreases this speed. Intriguingly, when deliberating between two visual options, saccade vigor to each option increases differentially, encoding their relative value. These results and others imply that vigor may serve as a new, real-time metric with which to quantify subjective utility, and that the control of movements may be an implicit reflection of the brain’s economic evaluation of the expected outcome.
Dan Goodman
Imperial college
October 25, 2023
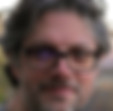
Multimodal units fuse-then-accumulate evidence across channels
We continuously detect sensory data, like sights and sounds, and use this information to guide our behaviour. However, rather than relying on single sensory channels, which are noisy and can be ambiguous alone, we merge information across our senses and leverage this combined signal. In biological networks, this process (multisensory integration) is implemented by multimodal neurons which are often thought to receive the information accumulated by unimodal areas, and to fuse this across channels; an algorithm we term accumulate-then-fuse. However, it remains an open question how well this theory generalises beyond the classical tasks used to test multimodal integration. Here, we explore this by developing novel multimodal tasks and deploying probabilistic, artificial and spiking neural network models. Using these models we demonstrate that multimodal units are not necessary for accuracy or balancing speed/accuracy in classical multimodal tasks, but are critical in a novel set of tasks in which we comodulate signals across channels. We show that these comodulation tasks require multimodal units to implement an alternative fuse-then-accumulate algorithm, which excels in naturalistic settings and is optimal for a wide class of multimodal problems. Finally, we link our findings to experimental results at multiple levels; from single neurons to behaviour. Ultimately, our work suggests that multimodal neurons may fuse-then-accumulate evidence across channels, and provides novel tasks and models for exploring this in biological systems.
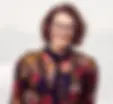
Kimberly Stachenfeld
Google Deep Mind
November 1, 2023
Prediction Models for Brains and Machines
Humans and animals learn and plan with flexibility and efficiency well beyond that of modern Machine Learning methods. This is hypothesized to owe in part to the ability of animals to build structured representations of their environments, and modulate these representations to rapidly adapt to new settings. In the first part of this talk, I will discuss theoretical work describing how learned representations in hippocampus enable rapid adaptation to new goals by learning predictive representations. I will also cover work extending this account, in which we show how the predictive model can be adapted to the probabilistic setting to describe a broader array of generalization results in humans and animals, and how entorhinal representations can be modulated to support sample generation optimized for different behavioral states. I will also talk about work applying this perspective to the deep RL setting, where we can study the effect of predictive learning on representations that form in a deep neural network and how these results compare to neural data. In the second part of the talk, I will overview some of the ways in which we have combined many of the same mathematical concepts with state-of-the-art deep learning methods to improve efficiency and performance in machine learning applications like physical simulation, relational reasoning, and design.
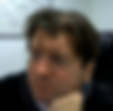
Viktor Jirsa
CNRS
November 8, 2023
Postponed to December 6
No seminar
November 15, 2023
Society for Neuroscience Meeting
No Seminar

Matthias Kaschube
Goethe-University, Frankfurt am Main
November 22, 2023
The Emergence of Cortical Representations
The internal and external world is thought to be represented by distributed patterns of cortical activity. The emergence of these cortical representations over the course of development remains an unresolved question. In this talk, I share results from a series of recent studies combining theory and experiments in the cortex of the ferret, a species with a well-defined columnar organization and modular network of orientation-selective responses in visual cortex. I show that prior to the onset of structured sensory experience, endogenous mechanisms set up a highly organized cortical network structure that is evident in modular patterns of spontaneous activity characterized by strong, clustered local and long-range correlations. This correlation structure is remarkably consistent across both sensory and association areas in the early neocortex, suggesting that diverse cortical representations initially develop according to similar principles. Next, I explore a classical candidate mechanism for producing modular activity – local excitation and lateral inhibition. I present the first empirical test of this mechanism through direct optogenetic cortical activation and discuss a plausible circuit implementation. Then, focusing on the visual cortex, I demonstrate that these endogenously structured networks enable orientation-selective responses immediately after eye opening. However, these initial responses are highly variable, lacking the reliability and low-dimensional structure observed in the mature cortex. Reliable responses are achieved after an experience-dependent co-reorganization of stimulus- evoked and spontaneous activity following eye opening. Based on these observations, I propose the hypothesis that the alignment between feedforward inputs and the recurrent network plays a crucial role in transforming the initially variable responses into mature and reliable representations.
Blake Bordelon
Harvard University
November 29, 2023

Mean Field Approaches to Learning Dynamics in Deep Networks
Deep neural network learning dynamics are very complex with large numbers of learnable weights and many sources of disorder. In this talk, I will discuss mean field approaches to analyze the learning dynamics of neural networks in large system size limits when starting from random initial conditions. The result of this analysis is a dynamical mean field theory (DMFT) where all neurons obey independent stochastic single site dynamics. Correlation functions (kernels) and response functions for the features and gradients at each layer can be computed self-consistently from these stochastic processes. Depending on the choice of scaling of the network output, the network can operate in a kernel regime or a feature learning regime in the infinite width limit. I will discuss how this theory can be used to analyze various learning rules for deep architectures (backpropagation, feedback alignment based rules, Hebbian learning etc), where the weight updates do not necessarily correspond to gradient descent on an energy function. I will then present recent extensions of this theory to residual networks at infinite depth and discuss the utility of deriving scaling limits to obtain consistent optimal hyperparameters (such as learning rate) across widths and depths. Feature learning in other types of architectures will be discussed if time permits. Lastly, I will discuss open problems and challenges associated with this theoretical approach to neural network learning dynamics.
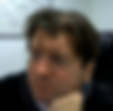
Viktor Jirsa
CNRS, Marseille
December 6, 2023
Digital Twins in Brain Medicine
Over the past decade we have demonstrated that the fusion of subject-specific structural information of the human brain with mathematical dynamic models allows building biologically realistic brain network models, which have a predictive value, beyond the explanatory power of each approach independently. The network nodes hold neural population models, which are derived using mean field techniques from statistical physics expressing ensemble activity via collective variables. Our hybrid approach fuses data-driven with forward-modeling-based techniques and has been successfully applied to explain healthy brain function and clinical translation including aging, stroke and epilepsy. Here we illustrate the workflow along the example of epilepsy: we reconstruct personalized connectivity matrices of human epileptic patients using Diffusion Tensor weighted Imaging (DTI). Subsets of brain regions generating seizures in patients with refractory partial epilepsy are referred to as the epileptogenic zone (EZ). During a seizure, paroxysmal activity is not restricted to the EZ, but may recruit other healthy brain regions and propagate activity through large brain networks. The identification of the EZ is crucial for the success of neurosurgery and presents one of the historically difficult questions in clinical neuroscience. The application of latest techniques in Bayesian inference and model inversion, in particular Hamiltonian Monte Carlo, allows the estimation of the EZ, including estimates of confidence and diagnostics of performance of the inference. The example of epilepsy nicely underwrites the predictive value of personalized large-scale brain network models. The workflow of end-to-end modeling is an integral part of the European neuroinformatics platform EBRAINS and enables neuroscientists worldwide to build and estimate personalized virtual brains.
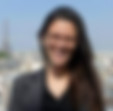
Laura Dugué
CNRS, Paris
December 13, 2023
Oscillatory Traveling Waves as a mechanism
for perception and attention
Brain oscillations have long been a topic of extensive research and debate regarding their potential functional role. Our research, and that of others, has shown that oscillations modulate perceptual and attentional performance periodically in time. Oscillations create periodic windows of excitability with more or less favorable periods recurring at particular phases of the oscillations. However, perception and attention emerge from systems not only operating in time, but also in space. In our current research we ask: how does the spatio-temporal organization of brain oscillations impact perception and attention? In this presentation, I will discuss our theoretical and experimental work on humans. We test the hypothesis that oscillations propagate over the cortical surface, so-called oscillatory Traveling Waves, allowing perception and attentional facilitation to emerge both in space and time.
December 20, 27 2023
CHRISTMAS & NEW YEAR VACATION
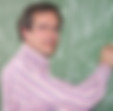
Marc Mézard
Bocconi University, Milano
January 3, 2024
Matrix Factorization with Neural Networks
The factorization of a large matrix into the product of two matrices is an important mathematical problem encountered in many tasks, ranging from dictionary learning to machine learning. Statistical physics can provide on the one hand theoretical limits on the possibility of factorizing matrices in the limit of infinite size, and also practical algorithms. While this program has been successful in the case of finite rank matrices, the regime of extensive rank (scaling linearly with the dimension of the matrix) turns out to be much harder. This talk will describe a new approach to matrix factorization that maps it to neural network models of associative memory: each pattern found in the associative memory corresponds to one factor of the matrix decomposition. A detailed theoretical analysis of this new approach shows that matrix factorization in the extensive rank regime is possible when the rank is below a certain threshold.
January 10, 2024
No seminar
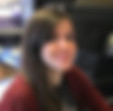
Ann Kennedy
Northwestern University
January 17, 2024
Neural computations underlying the regulation of motivated behavior
As we interact with the world around us, we experience a constant stream of sensory inputs, and must generate a constant stream of behavioral actions. What makes brains more than simple input-output machines is their capacity to integrate sensory inputs with an animal’s own internal motivational state to produce behavior that is flexible and adaptive. Working with neural recordings from subcortical structures involved in regulation of survival behaviors, we show how the dynamical properties of neural populations give rise to motivational states that change animal behavior on a timescale of minutes. We also show how neuromodulation can alter these dynamics to change behavior on timescales of hours to days.
N Alex Cayco Gajic
Ecole normale supérieure, Paris
January 24, 2024
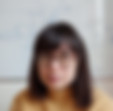
Discovering learning-induced changes in neural representations from large-scale neural data tensors
Learning induces changes in neural activity over slow timescales. These changes can be summarized by restructuring neural population data into a three-dimensional array or tensor, of size neurons by time points by trials. Classic dimensionality reduction methods often assume that neural representations are constrained to a fixed low-dimensional latent subspace. Consequently, this view does not capture how the latent subspace could evolve over learning, nor how high-dimensional neural activity could emerge over learning. Furthermore, the link between these empirically-observed changes in neural activity as a result of learning and circuit-level changes in recurrent dynamics is unclear. In this talk I will discuss our recent efforts towards developing dimensionality reduction and data-driven modeling methods based on tensors in order to identify how neural representations change over learning. First we introduce a new tensor decomposition, sliceTCA, which is able to disentangle latent variables of multiple covariability classes that are often mixed in neural population data. We demonstrate in three datasets that sliceTCA is able to capture more behaviorally-relevant information in neural data than previous methods. Second, to probe for how circuit-level changes in neural dynamics implement the observed changes in neural activity, we develop a data-driven RNN-based framework in which the recurrent connectivity is constrained to be low tensor rank. We demonstrate that such low tensor rank RNNs (ltrRNNs) are able to capture changes in neural geometry and dynamics in motor cortical data from a motor adaptation task. Together, both sliceTCA and ltrRNN demonstrate the utility of interpretable, tensor-based methods for discovery of learning-induced changes in neural representations directly from data.
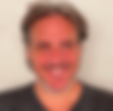.png)
Jonathan Kadmon
The Hebrew University
January 31, 2024
Neural mechanisms of adaptive behavior
Animals and humans rapidly adapt their behavior to dynamic environmental changes, such as predator threats or fluctuating food resources, often without immediate rewards. Existing literature posits that animals rely on internal representations of the environment, termed “beliefs”, for their decision policy. However, previous work ties belief updates to external reward signals, which does not explain adaptation in scenarios where trial-and-error approaches are inefficient or potentially perilous. In this work, we propose that the brain utilize dynamic representations that continuously infer the state of the environment, allowing it to update behavior rapidly. I will present a Bayesian theory for state inference in a partially observed Markov Decision Process with multiple interacting latent variables. Optimal behavior requires knowledge of hidden interactions between latent states. I will show that recurrent neural networks trained through reinforcement solve the task by learning the hidden interaction between latent states, and their activity encodes the dynamics of the optimal Bayesian estimators. The behavior of rodents trained on an identical task aligns with our theoretical model and neural network simulations, suggesting that the brain utilizes dynamic internal state representation and inference.
Nicholas Priebe
The University of Texas
at Austin
February 7, 2024
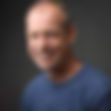.jpeg)
The origins of variable responses in neocortical neurons
I will discuss a collaborative project studying the origins of variable responses in neocortical neurons. The spiking responses of neocortical neurons are remarkably variable. Distinct patterns are observed when the same stimulus is presented in the sensory areas or when the same action is executed in motor areas. This is quantified across trials by measuring the Fano factor (FF) of the neuronal spike counts, which is generally near 1, consistent with spiking times following a noisy Poisson process.The two candidate sources for noise are the synaptic drive that converges on individual neurons or intrinsic transducing processes within neurons. To parse the relative contributions of these noise sources, we made whole-cell intracellular recordings from cortical slices and used in the whole cell dynamic clamp configuration while using dynamic clamp to injecting excitatory and inhibitory conductances previously recorded in vivo from visual cortical neurons (Tan et al. 2011). By controlling the conductance directly, we can test whether intrinsic processes contribute to poisson firing. We found that repeated injections of the same excitatory and inhibitory conductance evoked stereotypical spike trains, resulting in FF near 0.2. Varying the amplitude of both excitatory and inhibitory conductances changed the firing rate of recorded neurons but not the Fano factor. These records indicate that intrinsic processes do not contribute substantially to the Poisson spiking of cortical cells. Next, to test whether differences in network input are responsible for Poisson spike patterns, we examined spike trains evoked by injecting excitatory and inhibitory conductances recorded from different presentations of the same visual stimulus. These records exhibited different behaviors depending on whether the injected conductances were from visually-driven or spontaneous epochs: during visually-driven epochs, spiking responses were Poisson (FF near 1); during spontaneous epochs spiking responses were super-Poisson (FF above 1). Both of these observations are consistent with the quenching of variability by sensory stimulation or motor behavior (Churchland et al. 2010). We also found that excitatory conductances, in the absence of inhibition, are sufficient to generate spike trains with Poisson statistics. Our results indicate that the Poisson spiking emerges not from intrinsic sources but from differences in the synaptic drive across trials, the nature of this synaptic drive can alter the nature of variability, and that that excitatory input alone is sufficient to generate Poisson spiking.
Nader Nikbakht
MIT
February 14, 2024
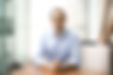
Thalamocortical dynamics in a complex learned behavior
Performing learned behaviors requires animals to produce precisely timed motor sequences. The underlying neuronal circuits must convert incoming spike trains into precisely timed firing to indicate the onset of crucial sensory cues or to carry out well-coordinated muscle movements. Birdsong is a remarkable example of a complex, learned and precisely timed natural behavior which is controlled by a brainstem-thalamocortical feedback loop. Projection neurons within the zebra finch cortical nucleus HVC (used as a proper name), produce precisely timed, highly reliable and ultra-sparse neural sequences that are thought to underlie song dynamics. However, the origin of short timescale dynamics of the song is debated. One model posits that these dynamics reside in HVC and are mediated through a synaptic chain mechanism. Alternatively, the upstream motor thalamic nucleus Uveaformis (Uva), could drive HVC bursts as part of a brainstem-thalamocortical distributed network. Using focal temperature manipulation we found that the song dynamics reside chiefly in HVC. We then characterized the activity of thalamic nucleus Uva, which provides input to HVC. We developed a lightweight (~1 g) microdrive for juxtacellular recordings and with it performed the very first extracellular single unit recordings in Uva during song. Recordings revealed HVC-projecting Uva neurons contain timing information during the song, but compared to HVC neurons, fire densely in time and are much less reliable. Computational models of Uva-driven HVC neurons estimated that a high degree of synaptic convergence is needed from Uva to HVC to overcome the inconsistency of Uva firing patterns. However, axon terminals of single Uva neurons exhibit low convergence within HVC such that each HVC neuron receives input from 2-7 Uva neurons. These results suggest that thalamus maintains sequential cortical activity during song but does not provide unambiguous timing information. Our observations are consistent with a model in which the brainstem-thalamocortical feedback loop acts at the syllable timescale (~100 ms) and does not support a model in which the brainstem-thalamocortical feedback loop acts at fast timescale (~10 ms) to generate sequences within cortex.
Itamar Landau
Stanford University
February 21, 2024
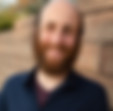
Random Matrix Theory and the Statistical Constraints of Inferring Population Geometry from Large-Scale Neural Recordings
Contemporary neuroscience has witnessed an impressive expansion in the number of neurons whose activity can be recorded simultaneously, from mere hundreds a decade ago to tens and even hundreds of thousands in recent years. With these advances, characterizing the geometry of population activity from large-scale neural recordings has taken center stage. In classical statistics, the number of repeated measurements is generally assumed to far exceed the number of free variables to be estimated. In our work, we ask a fundamental statistical question: as the number of recorded neurons grows, how are estimates of the geometry of population activity, for example, its dimensionality, constrained by the number of repeated experimental trials? Many neuroscience experiments report that neural activity is low-dimensional, with the dimensionality bounded as more neurons are recorded. We therefore begin by modeling neural data as a low-rank neurons-by-trials matrix with additive noise, and employ random matrix theory to show that under this hypothesis iso-contours of constant estimated dimensionality form hyperbolas in the space of neurons and trials -- estimated dimensionality increases as the product of neurons and trials. Interestingly, for a fixed number of trials, increasing the number of neurons improves the estimate of the high-dimensional embedding structure in neural space despite the fact that this estimation grows more difficult, by definition, with each neuron. While many neuroscience datasets report low-rank neural activity, a number of recent larger recordings have reported neural activity with "unbounded" dimensionality. With that motivation, we present new random matrix theory results on the distortion of singular vectors of high-rank signals due to additive noise and formulas for optimal denoising of such high-rank signals. Perhaps the most natural way to model neural data with unbounded dimensionality is with a power-law covariance spectrum. We examine the inferred dimensionality measured as the estimated power-law exponent, and surprisingly, we find that here too, under subsampling, the iso-contours of constant estimated dimensionality form approximate hyperbolas in the space of neurons and trials – indicating a non-intuitive but very real ompensation between neurons and trials, two very different experimental resources. We test these observations and verify numerical predictions on a number of experimental datasets, showing that our theory can provide a concrete prescription for numbers of neurons and trials necessary to infer the geometry of population activity. Our work lays a theoretical foundation for experimental design in contemporary neuroscience.
February 28 & March 6, 2024
Cosyne 2024
No Seminar
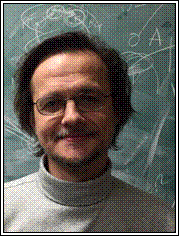
Paul Cisek
University of Montreal
March 13, 2024
Rethinking behavior in the light of evolution
In theoretical neuroscience, the brain is usually described as an information processing system that encodes and manipulates representations of knowledge to produce plans of action. This view leads to a decomposition of brain functions into putative processes such as object recognition, working memory, decision-making, action planning, etc., inspiring the search for the neural correlates of these processes. However, neurophysiological data do not support many of the predictions of these classic subdivisions. Instead, there is divergence and broad distribution of functions that should be unified, mixed representations combining functions that should be distinct, and a general incompatibility with the conceptual subdivisions posited by theories of information processing. In this talk, I will explore the possibility of resynthesizing a different set of functional subdivisions, guided by the growing body of data on the evolutionary process that produced the human brain. I will summarize, in chronological order, a proposed sequence of innovations that appeared in nervous systems along the lineage that leads from the earliest multicellular animals to humans. Along the way, functional subdivisions and elaborations will be introduced in parallel with the neural specializations that made them possible, gradually building up an alternative conceptual taxonomy of brain functions. These functions emphasize mechanisms for real-time interaction with the world, rather than for building explicit knowledge of the world, and the relevant representations emphasize pragmatic outcomes rather than decoding accuracy, mixing variables in the way seen in real neural data. I suggest that this alternative taxonomy may better delineate the real functional pieces into which the brain is organized, and can offer a more natural mapping between behavior and neural mechanisms.
Merav Stern
Rockefeller University
March 20, 2024
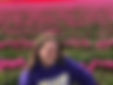
Conversions between space and time in network dynamics
by neural assemblies
The connectivity structure of many biological systems, including neural circuits, is highly non-uniform. Recent technologies allow detailed mapping of these irregularities, but our understanding of their effect on the overall circuit dynamics is still lacking. By developing complex system analytical tools that perform reduction of the network, I determine the impact of connectivity features on network dynamics. I will demonstrate the use of these tools on neural assemblies (clusters), a ubiquitous non-uniform structure in our brains. I will show how neural assemblies of different sizes naturally generate multiple timescales of activity spanning several orders of magnitude. I will demonstrate how the analytical theory we develop for rate networks, supported by spiking network simulations, reveals the dependency between neural timescales and assembly sizes and how new recordings of spontaneous activity from a million neurons support this analysis. I will also show how our model can naturally explain the particular long-tailed timescale distribution observed in the awake primate cortex. In olfactory cortex, neural assemblies represent odor stimuli. Previously, I showed how the diffuse recurrent excitation among these assemblies allows the conversion of time-encoded inputs from the bulb to spacial neural assembly representations in olfactory cortex. Here, I will show how changes in the dynamical properties of these assemblies alter both their timing response and properties of the time-encoded inputs via feedback. This demonstrates the role of neural assemblies in time-sensitive modulation needed for cognitive tasks, such as attention. Our results offer a biologically plausible mechanism of assemblies in network connectivity for explaining multiple puzzling dynamical phenomena: The ability of neural circuits to transform external simultaneous temporal fluctuations into spatial representations and alter them; and the ability of neuronal circuits to generate simultaneous temporal fluctuations across a large range of timescales;
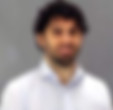
Lorenzo Fontolan
Université Aix-Marseille
March 27, 2024
Postponed to May 1st, 2024
Neural mechanisms of memory-guided behaviour
Persistent, stimulus-dependent neuronal activity has been observed in numerous brain areas during tasks that require the temporary maintenance of information. Several competing hypotheses for the neuronal mechanisms underlying persistent activity have been proposed. We have employed data-driven models in conjunction with optogenetic disruptions of neural circuits within memory-guided motor tasks. Our findings revealed a mechanism governed by dynamic attractors, pivotal in sustaining neuronal activity. This mechanism, shaped by time-varying inputs reflecting temporal predictions, is instrumental in regulating the impact of sensory information on the premotor cortex, thereby preserving memory traces from distracting stimuli. We then asked how persistent activity driven by attractor dynamics emerges during motor learning. It has been proposed that activity-dependent synaptic plasticity underpins motor learning, as it can reconfigure network architectures to produce the appropriate neural dynamics for specific behaviors. To verify this hypothesis, we investigated how the mouse premotor cortex acquires specific neural dynamics that govern the planning of movement at different stages of motor learning. We developed network models that replicated the effects of acute manipulations of synaptic plasticity. The models, which display attractor dynamics, also explain flexible behavior after learning has ended. By leveraging the model's predictions, we can formulate testable hypotheses regarding the distinct mechanisms governing movement planning at various stages of the learning process.
Manuel Beiran
Columbia University
April 3, 2024
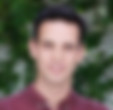
Prediction of neural activity in connectome-constrained
recurrent networks
In this talk, I will explain a theory of connectome-constrained neural networks in which a “student” networks is trained to reproduce the activity of a ground-truth “teacher”, representing a neural system for which a connectome is available. Unlike standard paradigms with unconstrained connectivity, here both networks have the same connectivity but they have different biophysical parameters, reflecting uncertainty in neuronal and synaptic properties. We find that the connectome is often insufficient to constrain the dynamics of networks that perform a specific task, illustrating the difficulty of inferring function from connectivity alone. However, recordings from a small subset of neurons can remove this degeneracy, producing dynamics in the student that agree with the teacher. Our theory can prioritize which neurons to record from to most efficiently unmeasured network activity. The analysis shows that the solution spaces of connectome-constrained and unconstrained models are qualitatively different, and provides a framework to determine when such models yield consistent dynamics.

Michael Buice
Allen Institute
April 10, 2024
Biologically motivated learning dynamics:
parallel architectures and nonlinear Hebbian plasticity.
Learning in biological systems takes place in contexts and with dynamics not often accounted for by simple models. I will describe the learning dynamics of two model systems that incorporate either architectural or dynamic constraints from biological observations. In the first case, inspired by the observed mesoscopic structure of the mouse brain as revealed by the Allen Mouse Brain Connectivity Atlas, as well as multiple examples of parallel pathways in mammalian brains, I present a mathematical analysis of learning dynamics in networks that have parallel computational pathways driven by the same cost function. We use the approximation of deep linear networks with large hidden layer sizes to show that, as the depth of the parallel pathways increases, different features of the training set (defined by the singular values of the input-output correlation) will typically concentrate in one of the pathways. This result is derived analytically and demonstrated with numerical simulation with both linear and non-linear networks. Thus, rather than sharing stimulus and task features across multiple pathways, parallel network architectures learn to produce sharply diversified representations with specialized and specific pathways, a mechanism which may hold important consequences for codes in both biological and artificial systems. In the second case, I discuss learning dynamics in a generalization of Hebbian rules and show that these rules allow a neuron to learn tensor decompositions of higher-order input correlations. Unlike the case of the Oja rule and PCA, the resulting learned representation is not unique but selects amongst the tensor eigenvectors according to initial conditions.
Tim O'Leary
University of Cambridge
April 17, 2024
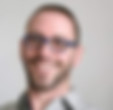
Flip flops and toggles for effective decision making in neural circuits
Neural computation is inextricably bound to decisions that must be made under time pressure and uncertainty. At the level of neural circuits, single neurons need to decide whether to spike. On longer timescales, the component circuitry needs to decide whether to reconfigure to store memories and adapt to novel situations. In this talk I will focus on two fun ideas in each of these contexts by showing how nonlinearities in neural components naturally form excitable switches that enable reliable decisions to be made in fluctuating environments. I will also issue propaganda that the kind of high level, cognitive faculties that we normally associate with decision making apply equally well and are understudied at the level of neural and synaptic populations.
April 24, 2024
Passover vacation
No seminar